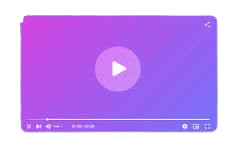
Human–AI feedback loops can amplify human’s biases
We begin by collecting human data in an emotion aggregation task in which human judgement is slightly biased. We then demonstrate that training an AI algorithm on this slightly biased dataset results in the algorithm not only adopting the bias but further amplifying it. Next, we show that when humans interact with the biased AI, their initial bias increases (Fig. 1a; human–AI interaction). This bias amplification does not occur in an interaction including only human participants (Fig. 1b; human–human interaction).
a, Human–AI interaction. Human classifications in an emotion aggregation task are collected (level 1) and fed to an AI algorithm (CNN; level 2). A new pool of human participants (level 3) then interact with the AI. During level 1 (emotion aggregation), participants are presented with an array of 12 faces and asked to classify the mean emotion expressed by the faces as more sad or more happy. During level 2 (CNN), the CNN is trained on human data from level 1. During level 3 (human–AI interaction), a new group of participants provide their emotion aggregation response and are then presented with the response of an AI before being asked whether they would like to change their initial response. b, Human–human interaction. This is conceptually similar to the human–AI interaction, except the AI (level 2) is replaced with human participants. The participants in level 2 are presented with the arrays and responses of the participants in level 1 (training phase) and then judge new arrays on their own as either more sad or more happy (test phase). The participants in level 3 are then presented with the responses of the human participants from level 2 and asked whether they would like to change their initial response. c, Human–AI-perceived-as-human interaction. This condition is also conceptually similar to the human–AI interaction condition, except participants in level 3 are told they are interacting with another human when in fact they are interacting with an AI system (input: AI; label: human). d, Human–human-perceived-as-AI interaction. This condition is similar to the human–human interaction condition, except that participants in level 3 are told they are interacting with AI when in fact they are interacting with other humans (input: human; label: AI). e, Level 1 and 2 results. Participants in level 1 (green circle; n = 50) showed a slight bias towards the response more sad. This bias was amplified by AI in level 2 (blue circle), but not by human participants in level 2 (orange circle; n = 50). The P values were derived using permutation tests. All significant P values remained significant after applying Benjamini–Hochberg false discovery rate correction at α = 0.05. f, Level 3 results. When interacting with the biased AI, participants became more biased over time (human–AI interaction; blue line). In contrast, no bias amplification was observed when interacting with humans (human–human interaction; orange line). When interacting with an AI labelled as human (human–AI-perceived-as-human interaction; grey line) or humans labelled as AI (human–AI-perceived-as-human interaction; pink line), participants’ bias increased but less than for the human–AI interaction (n = 200 participants). The shaded areas and error bars represent s.e.m.
Humans exhibit a small judgement bias
Fifty participants performed an emotion aggregation task (adapted from refs. 41,42,43,44). On each of 100 trials, participants were presented briefly (500 ms) with an array of 12 faces and were asked to report whether the mean emotion expressed by the faces in the array was more sad or more happy (Fig. 1a; level 1). The faces were sampled from a dataset of 50 morphed faces, created by linearly interpolating between sad and happy expressions (Methods). Based on the morphing ratio, each face was ranked from 1 (100% sad face) to 50 (100% happy face). These rankings were closely associated with participants’ own rankings of each face when observed one by one (b = 0.8; t(50) = 26.25; P < 0.001; see Supplementary Results). We created 100 unique arrays of 12 faces for each participant. The average ranking of the 12 faces in half of the arrays was smaller than 25.5 (thus, the array was more sad) and greater than 25.5 in the other half (thus the array was more happy).
Bias in this task was defined as the difference between the average responses of a participant across all trials and the actual average. The actual average in the task was 0.5, as responses were coded as either 1 (more sad) or 0 (more happy), and exactly half of the trials were more sad and half were more happy. Mathematically, the bias is expressed as:
$${{\rm{Bias}}}=\frac{1}{n}\mathop{\sum }\limits_{i=1}^{n}{C}_{i}-0.5$$
Where n denotes the total number of data points and Ci denotes the classification assigned to each data point (Ci = 1 for a more sad classification and Ci = 0 for a more happy classification). A positive bias indicates a tendency towards classifying responses as more sad, whereas a negative bias suggests a leaning towards classifying responses as more happy. For example, if a participant were to classify 0.7 of the arrays as more sad, their bias would be 0.7 − 0.5 = 0.2, whereas if they were to classify 0.3 of the arrays as more sad, their bias would be 0.3 − 0.5 = −0.2.
Consistent with previous studies showing that interpretation of an ambiguous valence is more likely to be negative under short encoding times45,46, participants showed a slight but significant tendency to report that the faces were more sad. In particular, they categorized 53.08% of the arrays as more sad, which is a greater proportion than would be expected by chance (permutation test against 50%: P = 0.017; d = 0.34; 95% confidence interval (CI)more sad = 0.51 to 0.56; green circle in Fig. 1e; see also Supplementary Results for estimation of the bias by psychometric function analysis). The bias was much larger in the first block than subsequent blocks (Mblock 1 = 56.72%; Mblocks 2–4 = 51.87%; permutation test comparing the first block with the rest: P = 0.002; d = 0.46; 95% CI = 0.02 to 0.08), suggesting that the participants corrected their bias over time.
AI trained on biased human data amplifies the bias
Next, we used a CNN7 to classify each array of faces into more happy or more sad. As detailed below, the CNN amplified the classification bias observed in the human participants (see Methods for further details of the model).
First, to test the accuracy of the model, we trained it on the 5,000 arrays that were presented to the participants in level 1 (5,000 arrays = 50 participants × 100 arrays), with class labels based on the objective ranking scores of the arrays (that is, not the human labels). The model was then evaluated on a 300 out-of-sample test set and showed a classification accuracy of 96%, suggesting that it was highly accurate and did not show a bias if trained on non-biased data (see Table 1). Next, we trained the model on class labels defined based on the human classification (5,000 samples of arrays; Fig. 1a) and evaluated it on 300 arrays in an out-of-sample test set. The model classified the average emotion as more sad in 65.33% of the cases, despite only 50% of the arrays being more sad. This number was significantly greater than would be expected by chance (permutation test against 50%: P < 0.001; 95% CImore sad = 0.60 to 0.71; blue circle in Fig. 1e) and significantly greater than the bias observed in the human data (level 1), which was only 53% (permutation test: P < 0.001; d = 1.33; 95% CI = 0.09 to 0.14; Fig. 1e). In other words, the AI algorithm greatly amplified the human bias embedded in the data it was trained on. Similar results were obtained for CNNs with different architectures, including ResNet50 (ref. 47; see Supplementary Results).
A possible reason for the bias amplification of the AI is that it exploits biases in the data to improve its prediction accuracy. This should happen more when the data are noisy or inconsistent. To test this hypothesis, we retrained the model with two new sets of labels. First, we used non-noisy labels (that is, based on the objective ranking scores of the arrays), but induced a minor bias by switching 3% of the labels. Thus, 53% of the labels were classified as more sad. Second, we used very noisy labels (random labels), in which we also induced a 3% bias. If the bias amplification were due to noise, the bias of the latter model should be higher than that of the former. The results confirmed this hypothesis (Table 1): the average bias of the model trained on the accurate labels with a minor bias was exactly 3%, whereas the average bias of the model trained on the random labels with a bias of 3% was 50% (that is, the model classified 100% of arrays as more sad). These results indicate that the bias amplification of the CNN model is related to the noise in the data.
Interaction with biased AI increases human bias
Next, we set out to examine whether interacting with the biased AI algorithm would alter human judgements (Fig. 1a; level 3). To this end, we first measured participants’ baseline performance on the emotion aggregation task for 150 trials, so that we could compare their judgements after interacting with the AI versus before. As in level 1, we found that participants had a small bias at first (Mblock 1 = 52.23%), which decreased in subsequent blocks, (Mblocks 2–5 = 49.23%; permutation test testing the first block against the rest of the blocks: P = 0.03; d = 0.31; 95% CI = 0.01 to 0.06). The next question was whether interacting with AI would cause the bias to reappear in humans and perhaps even increase.
To test this hypothesis, on each of 300 trials, participants first indicated whether the array of 12 faces was more sad or more happy. They were then presented with the response of the AI to the same array (participants were told that they “will be presented with the response of an AI algorithm that was trained to perform the task”). They were then asked whether they would like to change their initial response or not (that is, from more sad to more happy or vice versa). The participants changed their response on 32.72% (±2.3% s.e.) of the trials in which the AI provided a different response and on 0.3% (±0.1% s.e.) of the trials in which the AI provided the same response as they did (these proportions are significantly different: permutation test: P < 0.001; d = 1.97; 95% CI = 0.28 to 0.37). Further study (Supplementary Experiment 1) showed that when not interacting with any associate, participants changed their decisions only on 3.97% of trials, which was less than when interacting with a disagreeing AI (permutation test: P < 0.001; d = −2.53; 95% CI = −0.57 to −0.42) and more than when interacting with an agreeing AI (permutation test: P < 0.001; d = 0.98; 95% CI = 0.02 to 0.05).
The primary question of interest, however, was not whether participants changed their response after observing the AI’s response. Rather, it was whether over time their own response regarding an array (before observing the AI’s response to that specific array) became more and more biased due to previous interactions with the AI. That is, did participants learn to become more biased over time?
Indeed, whereas in the baseline blocks participants classified on average only 49.9% (±1.1% s.e.) of the arrays as more sad, when interacting with the AI this rate increased significantly to 56.3% (±1.1% s.e.; permutation test for interaction blocks against baseline: P < 0.001; d = 0.84; 95% CImore sad = 0.54 to 0.59). The learned bias increased over time: in the first interaction block it was only 50.72%, whereas in the last interaction block it was 61.44%. This increase in bias was confirmed by a linear mixed model predicting a higher rate of more sad classifications as the block number (a fixed factor) increased, with random intercepts and slopes at the participant level (b = 0.02; t(50) = 6.23; P < 0.001; Fig. 1f).
These results demonstrate an algorithmic bias feedback loop; training an AI algorithm on a set of slightly biased human data results in the algorithm amplifying it. Subsequent interactions of other humans with this algorithm further increase the humans’ initial bias levels, creating a feedback loop.
Human–human interactions did not amplify bias
Next, we investigated whether the same degree of bias contagion occurs in interactions involving only humans. To this end, we used the same interaction structure as above, except the AI system was replaced with human participants (Fig. 1b).
Humans exhibit a small judgement bias
The responses used in the first level of the human–human interaction were the same as those used in the human–AI interaction described above.
Humans trained on human data do not amplify bias
Conceptually similar to AI algorithm training, here we aimed to train humans on human data (Fig. 1b; level 2). The participants were presented with 100 arrays of 12 faces. They were told they would be presented with the responses of other participants who performed the task before. For each of the 100 arrays, they observed the response of a pseudo-randomly selected participant from level 1 (see Methods for further details). Thereafter, they judged ten new arrays on their own (as either more sad or more happy). To verify that the participants attended to the responses of the other level 1 participants, they were asked to report them on 20% of the trials (randomly chosen). Participants who gave an incorrect answer on more than 10% of the trials (and thus were not attending the task; n = 14), were excluded from the experiment.
Participants characterized the arrays as more sad 54.8% of the time, which is more than would be expected by chance (permutation test against 50%: P = 0.007; d = 0.41; 95% CImore sad = 52 to 58%). Critically, this result did not differ from that of level 1 human participants (permutation test level 1 humans versus level 2 humans: P = 0.43; d = 0.11; 95% CI = −0.02 to 0.06; Fig. 1e), but was significantly lower than for the AI algorithm, which characterized 65.13% of the arrays as more sad (permutation test level 2 humans against level 2 AI: P < 0.001; d = 0.86; 95% CI = −0.07 to −0.013; Fig. 1e). This difference was unlikely to have been driven by variations in training sample sizes, as the effect was observed even when AI and human participants were trained on identical datasets (Supplementary Experiment 2). Furthermore, the results were generalized to a different training method, in which participants were incentivized to actively predict the responses of other participants (Supplementary Experiment 3).
In conclusion, unlike the AI, human bias was not amplified after being trained on biased human data. This is not surprising, as the level of bias participants in level 2 naturally exhibit is probably the same as the one they were trained on. Moreover, unlike AI systems, humans base their judgements on factors that go beyond the training session, such as previous experiences and expectations.
Human–human interaction does not increase bias
Next, we exposed a new pool of participants (n = 50) to the judgements of humans from level 2. The task and analysis were identical to those described for level 3 of the human–AI interaction (except, of course, participants were interacting with humans, which they were made aware of; Fig. 1b).
Before being exposed to the other human’s response, participants completed five baseline blocks. As in levels 1 and 3 (human–AI interaction), participants showed a significant bias during the first block (Mblock 1 = 53.67%) which disappeared over time (Mblocks 2–5 = 49.87%; permutation test for the first baseline block against the rest of the baseline blocks: P = 0.007; d = 0.40; 95% CI = 0.01 to 0.06).
Next, participants interacted with other human participants (human–human interaction; level 2). As expected, participants changed their classification more when the other participants disagreed with them (11.27 ± 1.4% s.e.) than when they agreed with them (0.2 ± 0.03% s.e.) (permutation test comparing the two: P < 0.001; d = 1.11; 95% CI = 0.08 to 0.14) and less than when interacting with a disagreeing AI (which was 32.72%; permutation test comparing the response change when interacting with a disagreeing AI compared with interacting with a disagreeing human: P < 0.001; d = 1.07; 95% CI = 0.16 to 0.27).
Importantly, there was no evidence of learned bias in the human–human interaction (Fig. 1f). Classification rates were no different when interacting with other humans (Mmore sad = 51.45 ± 1.3% s.e.) than baseline (50.6 ± 1.3% s.e.) (permutation test for interaction blocks against baseline: P = 0.48; d = 0.10; 95% CImore sad = −0.01 to 0.03) and did not change over time (b = 0.003; t(50) = 1.1; P = 0.27).
Taken together, these results indicate that human bias is significantly amplified in a human–AI interaction, more so than in interactions between humans. These findings suggest that the impact of biased AI systems extends beyond their own biased judgement to their ability to bias human judgement. This raises concerns for human interactions with potentially biased algorithms across different domains.
AI’s output and human perception of AI shape its influence
A question that arises is whether participants became more biased when interacting with the AI system compared with humans because the AI provided more biased judgements, because they perceived the AI system differently than other humans, or both. To address this question, we ran two additional iterations of the experiment. In the first iteration (AI perceived as human), participants interacted with an AI system but were told they were interacting with another human participant (Fig. 1c). In the second iteration (human perceived as AI), participants interacted with an AI system but were told they were interacting with another human participant (Fig. 1d).
To this end, new pools of participants (n = 50 per condition) were recruited. First, they performed the baseline test described above and then they interacted with their associate (level 3). When interacting with the AI (which was believed to be a human) participants’ bias increased over time: in the first interaction block it was only 50.5%, whereas in the last interaction block it was 55.28% (Fig. 1f). The increase in bias across blocks was confirmed by a linear mixed model predicting a higher rate of more sad classifications as the block number (a fixed factor) increased, with random intercepts and slopes at the participant level (b = 0.01; t(50) = 3.14; P < 0.001). Similar results were obtained for the human–human-perceived-as-AI interaction. The bias increased across blocks (from 49.0% in the first block to 54.6% in the last), as was confirmed by a linear mixed model (b = 0.01; t(50) = 2.85; P = 0.004; Fig. 1f). In both cases, the bias was greater than at baseline (human–AI perceived as human: Mbias = 3.85 (permutation test comparing with baseline: P = 0.001; d = 0.49; 95% CI = 0.02 to 0.06); human–human perceived as AI: Mbias = 2.49 (permutation test comparing with baseline: P = 0.04; d = 0.29; 95% CI = 0.01 to 0.05)).
Was the induced bias a consequence of the type of input (AI versus human) or the perception of that input (perceived as AI versus perceived as human)? To investigate this, we submitted the induced bias scores (the percentage of more sad judgements minus the baseline percentage of more sad judgements) into a 2 (input: AI versus human) × 2 (label: AI versus human) analysis of variance (ANOVA) with time (blocks 1–6) as a covariate (Fig. 1f). The results revealed interactions between input and time (F(4.55, 892.35) = 3.40; P = 0.006) and between label and time (F(4.55, 892.35) = 2.65; P = 0.026). In addition, there were main effects of input (F(1, 196) = 9.45; P = 0.002) and time (F(4.55, 892.35) = 14.80; P < 0.001). No other effects were significant (all P values > 0.06). Thus, as illustrated in Fig. 1f, both the AI’s input and its label contributed to enhanced bias in humans over time.
Finally, we assessed the rate of decision changes among participants. Participants were more likely to change their classification when their associate disagreed with them. In human–AI-perceived-as-human interactions, decision changes occurred at a rate of 16.84% (±1.2% s.e.) when there was a disagreement, compared with a mere 0.2% (±0.05% s.e.) when agreeing (permutation test comparing the two: P < 0.001; d = 1.22; 95% CI = 0.13 to 0.20). Similarly, for the human–human-perceived-as-AI condition, decision changes were observed in 31.84% (±2.5% s.e.) when disagreement existed, compared with 0.4% (±0.1% s.e.) in cases of agreement (permutation test comparing the two: P < 0.001; d = 1.7; 95% CI = 0.26 to 0.36).
To quantify the effects of input and label on decision changes in cases of disagreement, we submitted the percentage of decision change into a 2 (input: AI versus human) × 2 (label: AI versus human) ANOVA with time (blocks 1–6) as a covariate. The results revealed that both the AI’s input (F(1, 196) = 7.05; P = 0.009) and its label (F(1, 196) = 76.30; P < 0.001) increased the likelihood of a decision change. These results remained consistent after applying Welch’s correction to address violations of the homogeneity of variance assumption: for AI’s input F(1, 197.92) = 5.11 and P = 0.02 and for AI’s label F(1, 175.57) = 74.21 and P < 0.001. All other main effects and interactions were not significant (all P values > 0.13).
Biased algorithms bias decisions, whereas accurate ones improve them
Next, we sought to generalize the above results to different types of algorithm and domain. In particular, we aimed to mimic a situation in which humans are not a priori biased, but rather AI bias emerges for other reasons (for example, if it was trained on unbalanced data). To this end, we employed a variant of the random dot kinematogram (RDK) task48,49,50,51, in which participants were presented with an array of moving dots and asked to estimate the percentage of dots that moved from left to right on a scale ranging from 0% (no dots moved from left to right) to 100% (all dots moved from left to right). To estimate baseline performance, participants first performed the RDK task on their own for 30 trials and reported their confidence on a scale ranging from not confident at all to very confident (Fig. 2a). Across trials, the actual average percentage of dots that moved rightward was 50.13 ± 20.18% (s.d.), which was not significantly different from 50% (permutation test against 50%: P = 0.98; d = 0.01; 95% CI = 42.93 to 57.33%), and the average confidence was 0.56 ± 0.17 (s.d.).
a, Baseline block. Participants performed the RDK task, in which an array of moving dots was presented for 1 s. They estimated the percentage of dots that moved from left to right and reported their confidence. b, Algorithms. Participants interacted with three algorithms: accurate (blue distribution), biased (orange distribution) and noisy (red distribution). c, Interaction blocks. Participants provided their independent judgement and confidence (self-paced) and then observed their own response and a question mark where the AI algorithm response would later appear. Participants were asked to assign weights to their response and the response of the algorithm (self-paced). Thereafter, the response of the algorithm was revealed (2 s). Note that the AI algorithm’s response was revealed only after the participants indicated their weighting. As a result, they had to rely on their global evaluation of the AI based on previous trials. d, AI-induced bias. Interacting with a biased AI resulted in significant human bias relative to baseline (P values shown in red) and relative to interactions with the other algorithms (P values shown in black; n = 120). e, When interacting with a biased algorithm, AI-induced bias increases over time (n = 50). f, AI-induced accuracy change. Interacting with an accurate AI resulted in a significant increase in human accuracy (that is, reduced error) relative to baseline (P values shown in red) and relative to interactions with the other algorithms (P values shown in black; n = 120). g, When interacting with an accurate algorithm, AI-induced accuracy increases over time (n = 50). h,i, Participants perceived the influence of the accurate algorithm on their judgements to be greatest (h; n = 120), even though the actual influence of the accurate and biased algorithms was the same (i; n = 120). The thin grey lines and circles correspond to individual participants. In d and f, the circles correspond to group means, the central lines represent median values and the bottom and top edges are the 25th and 75th percentiles, respectively. In e and g, the error bars represent s.e.m. The P values were derived using permutation tests. All significant P values remained significant after applying Benjamini–Hochberg false discovery rate correction at α = 0.05.
To examine whether and how different algorithmic response patterns affect human decision-making, we used three simple algorithms: accurate, biased and noisy. The accurate algorithm always indicated the correct percentage of dots that moved from left to right (Fig. 2b; blue distribution). The biased algorithm provided systematically upward biased estimates of dots that moved to the right (Fig. 2b; orange distribution; Mbias = 24.96). The noisy algorithm provided responses that were equal to those of the accurate algorithm plus Gaussian noise (s.d. = 30; Fig. 2b; red distribution). The biased and noisy algorithms had the same absolute error (Methods). The algorithms used here were hard coded to allow full control over their responses.
On each trial, participants first provided their judgement and confidence and then observed their own response and a question mark where the algorithm response would later appear (Fig. 2c). They were asked to assign weight to their own response and to that of the algorithm on a scale ranging from 100% you to 100% AI (Methods). Thus, if a participant assigned a weight of w to their own response, the final joint decision would be:
$$\begin{array}{l}{{\rm{Final}}\; {\rm{joint}}\; {\rm{decision}}}\\=w \times({{\rm{participant}}}\mbox{‘}{{\rm{s}}\; {\rm{response}}})+(1-w)\times\,({{\rm{AI}}}\mbox{‘}{{\rm{s}}\; {\rm{response}}})\end{array}$$
This weighting task is analogous to the change decision task in experiment 1; however, here we used a continuous scale instead of a binary choice, allowing us to obtain a finer assessment of participants’ judgements.
After participants provided their response, the response of the AI algorithm was revealed (Fig. 2c). Note that the AI algorithm response was exposed only after the participants indicated their weighting. This was done to prevent participants from relying on the concrete response of the algorithm on a specific trial, instead making them rely on their global evaluation of the algorithm. The participants interacted with each algorithm for 30 trials. The order of the algorithms (bias, noisy or accurate) was counterbalanced.
Bias in the RDK task was defined as follows:
$${\rm{Bias}}=\frac{{\sum }_{i=1}^{n}({\rm{Participant}}\mbox{‘}{\rm{s}}\,{\rm{response}}_{i}-{\rm{Evidence}}_{i})}{n}$$
where i and n correspond to the index of the present trial and the total number of trials, respectively. Evidence corresponds to the percentage of dots that moved rightward in the i-th trial. To compute AI-induced bias in participants, we subtracted the participant’s bias in the baseline block from the bias in the interaction blocks.
$${{\rm{AI}}{\mbox{-}}{\rm{induced}}\; {\rm{bias}}}={{\rm{Bias}}}_{{{\rm{AI}}\; {\rm{interaction}}\; {\rm{blocks}}}}-{{\rm{Bias}}}_{{{\rm{baseline}}}}$$
At the group level, no systematic bias in baseline responses was detected (mean response at baseline = 0.62; permutation test against 0: P = 0.28; d = 0.1; 95% CI = −0.48 to 1.76).
To define accuracy, we first computed an error score for each participant:
$${\rm{Error}}=\frac{{\sum }_{i=1}^{n}|{\rm{Participant}}\mbox{‘} s\,{\rm{response}}_{i}-{\rm{Evidence}}_{i}|}{n}$$
Then, this quantity was subtracted from the error score in the baseline block, indicating changes in accuracy.
$${{\rm{AI}}{\mbox{-}}{\rm{induced}}\; {\rm{accuracy}}\; {\rm{change}}}={{\rm{Error}}}_{{{\rm{baseline}}}}-{{\rm{Error}}}_{{{\rm{AI}}\; {\rm{interaction}}\; {\rm{blocks}}}}$$
That is, if errors when interacting with the AI (second quantity) were smaller than baseline errors (first quantity), the change would be positive, indicating that participants became more accurate. However, if errors when interacting with the AI (second quantity) were larger than during baseline (first quantity), the change would be negative, indicating that participants became less accurate when interacting with the AI.
The results revealed that participants became more biased (towards the right) when interacting with the biased algorithm relative to baseline performance (Mbias (biased AI) = 2.66 and Mbias (baseline) = 0.62; permutation test: P = 0.002; d = 0.28; 95% CI = 0.76 to 3.35; Fig. 2d) and relative to when interacting with the accurate algorithm (Mbias (accurate AI) = 1.26; permutation test: P = 0.006; d = 0.25; 95% CI = 0.42 to 2.37; Fig. 2d) and the noisy algorithm (Mbias (noisy AI) = 1.15; permutation test: P = 0.006; d = 0.25; 95% CI = 0.44 to 2.56; Fig. 2d). No differences in bias were found between the accurate and noisy algorithms, nor when interacting with these algorithms relative to baseline performance (all P values > 0.28). See also Supplementary Results for analysis of the AI-induced bias on a trial-by-trial basis.
The AI-induced bias was replicated in a follow-up study (n = 50; Methods) in which participants interacted exclusively with a biased algorithm across five blocks (Mbias = 5.03; permutation test: P < 0.001; d = 0.72; 95% CI = 3.14 to 6.98; Fig. 2e). Critically, we found a significant linear relationship over time (b = 1.0; t(50) = 2.99; P = 0.004; Fig. 2e), indicating that the more participants interacted with the biased algorithm, the more biased their judgements became. The learning of bias induced by the AI was also supported by a computational learning model (Supplementary Models).
Interaction with the accurate algorithm increased the accuracy of participants’ independent judgements compared with baseline performance (Merrors (accurate AI) = 13.48, Merrors (baseline) = 15.03 and Maccuracy change (accurate AI) = 1.55; permutation test: P < 0.001; d = 0.32; 95% CI = 0.69 to 2.42; Fig. 2f) and compared with when interacting with the biased algorithm (Merrors (biased AI) = 14.73 and Maccuracy change (biased AI) = 0.03; permutation test: P < 0.001; d = 0.33; 95% CI = 0.58 to 1.94; Fig. 2f) and the noisy algorithm (Merrors (noisy AI) = 14.36 and Maccuracy change (noisy AI) = 0.67; permutation test: P = 0.01; d = 0.22; 95% CI = 0.22 to 1.53; Fig. 2f). No differences in induced accuracy change were found between the biased and noisy algorithms, nor were there differences in errors when interacting with these algorithms relative to baseline performance (all P values > 0.14; Fig. 2f).
The AI-induced accuracy change was replicated in a follow-up study (n = 50; Methods) in which participants interacted exclusively with an accurate algorithm across five blocks (Maccuracy change = 3.55; permutation test: P < 0.001; d = 0.64; 95% CI = 2.14 to 5.16; Fig. 2g). Critically, we found a significant linear relationship for the AI-induced accuracy change over time (b = 0.84; t(50) = 5.65; P < 0.001; Fig. 2g), indicating that the more participants interacted with the accurate algorithm, the more accurate their judgements became. For participants’ confidence rating and weight assignment decisions, see Supplementary Results.
Importantly, the increase in accuracy when interacting with the accurate AI could not be attributed to participants copying the algorithm’s accurate response, not could the increased bias when interacting with the biased algorithm be attributed to participants copying the algorithm’s biased responses. This is because we purposefully designed the task such that participants would indicate their judgements on each trial before they observed the algorithm’s response. Instead, the participants learned to provide more accurate judgements in the former case and learned to provide more biased judgements in the latter case.
Participants underestimate the biased algorithm’s impact
We sought to explore whether participants were aware of the substantial influence the algorithms had on them. To test this, participants were asked to evaluate to what extent they believed their responses were influenced by the different algorithms they interacted with (Methods). As shown in Fig. 2h, participants reported being more influenced by the accurate algorithm compared with the biased one (permutation test: P < 0.001; d = 0.57; 95% CI = 0.76 to 1.44) and the noisy one (permutation test: P < 0.001; d = 0.58; 95% CI = 0.98 to 1.67). No significant difference was found between how participants perceived the influence of the biased and noisy algorithms (permutation test: P = 0.11; d = 0.15; 95% CI = −0.05 to 0.52).
In reality, however, the magnitude by which they became more biased when interacting with a biased algorithm was equal to the magnitude by which they became more accurate when interacting with an accurate algorithm. We quantified influence using two different methods (Methods) and both revealed the same result (Fig. 2i; z-scoring across algorithms: permutation test: P = 0.90; d = −0.01; 95% CI = −0.19 to 0.17; as a percentage difference relative to baseline: permutation test: P = 0.89; d = −0.02; 95% CI = −1.44 to 1.90).
These results show that in different paradigms, and under different response protocols, interacting with a biased algorithm biases participants’ independent judgements. Moreover, interacting with an accurate algorithm increased the accuracy of participants’ independent judgements. Strikingly, the participants were unaware of the strong effect that the biased algorithm had on them.
Real-world generative AI-induced bias in social judgements
Thus far, we have demonstrated that interacting with biased algorithms leads to more biased human judgements in perceptual and emotion-based tasks. These tasks allowed for precise measurements and facilitated our ability to dissociate effects. Next, we aimed to generalize these findings to social judgements by using AI systems commonly employed in real-world settings, thereby increasing the ecological validity of our results52,53,54 (see also Supplementary Experiment 5 for a controlled experiment examining a social judgement task). To this end, we examined changes to human judgements following interactions with Stable Diffusion—a widely used generative AI system designed to create images based on textual prompts55.
Recent studies have reported that Stable Diffusion amplifies existing social imbalances. For example, it over-represents White men in high-power and high-income professions compared with other demographic groups30,56. Such biases can stem from different sources, including problematic training data and/or flawed content moderation techniques30. Stable Diffusion outputs are used in diverse applications, such as videos, advertisements and business presentations. Consequently, these outputs have the potential to impact humans’ belief systems, even when an individual does not directly interact with the AI system but merely observes its output (for example, on social media, in advertisements or during a colleague’s presentation). Here, we test whether interacting with Stable Diffusion’s outputs increases bias in human judgement.
To test this, we first prompted Stable Diffusion to create: “A color photo of a financial manager, headshot, high-quality” (Methods). As expected, the images produced by Stable Diffusion over-represented White men (85% of images) relative to their representation in the population. For example, in the United States only 44.3% of financial managers are men57, of whom a fraction are White, and in the United Kingdom only about half are men58, of whom a fraction are White. In other Western countries the percentage of financial managers who are White men is also less than 85% and in many non-Western countries the numbers are probably even lower.
Next, we conducted an experiment (n = 100) to examine how participants’ judgements about who is most likely to be a financial manager would alter after interactions with Stable Diffusion. To this end, before and after interacting with Stable Diffusion, participants completed 100 trials. On each trial, they were presented with images of six individuals from different race and gender groups: (1) White men; (2) White women; (3) Asian men; (4) Asian women; (5) Black men; and (6) Black women (see Fig. 3a; stage 1; baseline). The images were taken from the Chicago Face Database59 and were balanced in terms of age, attractiveness and racial prototypicality (Methods). On each trial, participants were asked: “which person is most likely to be a financial manager?”. They responded by clicking on one of the images. Before this, participants were provided with a definition of financial manager (Methods). We were interested in whether participants’ responses would gravitate towards White men after interacting with Stable Diffusion outputs.
a, Experimental design. The experiment consisted of three stages. In stage 1, participants were presented with images featuring six individuals from different race and gender groups: a White man, a White woman, an Asian man, an Asian woman, a Black man and a Black woman. On each trial, participants selected the person who they thought was most likely to be a financial manager. In stage 2, for each trial, three images of financial managers generated by Stable Diffusion were randomly chosen and presented to the participants. In the control condition, participants were presented with three images of fractals instead. In stage 3, participants repeated the task from stage 1, allowing measurement of the change in participants’ choices before versus after exposure to the AI-generated images. b, The results revealed a significant increase in participants’ inclination to choose White men as financial managers after being exposed to AI-generated images, but not after being exposed to fractal neutral images (control). The error bars represent s.e.m. Face stimuli in a reproduced from ref. 59 under a Creative Commons licence CC BY 4.0.
Before interacting with Stable Diffusion, participants selected White men, White women, Asian men, Asian women, Black men and Black women 32.36, 14.94, 14.40, 20.24, 6.64 and 11.12% of the time, respectively. Although there is no definitive ground truth here, based on demographic data, White men is estimated not to be a normative response (for details, see Supplementary Results). Next, participants were exposed to the outputs of Stable Diffusion (see Fig. 3a; stage 2; exposure). Specifically, participants were told that they would be shown three images of financial managers generated by AI (Stable Diffusion) and received a brief explanation about Stable Diffusion (Methods). Then, on each trial, participants viewed three images of financial managers that were randomly chosen from those generated by Stable Diffusion for 1.5 s. This brief exposure time mimics common real-world interaction with AI-generated content on platforms such as social media, news websites and advertisements. Such encounters are often brief, with users rapidly scrolling through content. For example, the average viewing time for images on mobile devices is 1.7 s (ref. 60).
In stage 3 (Fig. 3a; stage 3; post-exposure), participants repeated the task from stage 1. The primary measure of interest was the change in participants’ judgements. The data were analysed using a mixed model multinomial logistic regression with exposure (before versus after exposure to AI images) as a fixed factor, with random intercepts and slopes at the participant level. This model was chosen because the dependent variable involved a choice from six distinct and unordered categories (see Supplementary Results for an alternative analysis).
The findings revealed a significant effect for exposure (F(5, 62) = 5.89; P < 0.001; Fig. 3b), indicating that exposure to the AI images altered human judgements. In particular, exposure increased the likelihood of choosing White men as financial managers (Mbefore exposure = 32.36%; Mafter exposure = 38.20%) compared with White women (Mbefore exposure = 14.94%; Mafter exposure = 14.40%; b = 0.26; t = 2.08; P = 0.04; 95% CI = 0.01 to 0.50), Asian women (Mbefore exposure = 20.24%; Mafter exposure = 17.14%; b = 0.47; t = 3.79; P < 0.001; 95% CI = 0.22 to 0.72), Black men (Mbefore exposure = 6.64%; Mafter exposure = 5.62%; b = 0.65; t = 3.04; P = 0.004; 95% CI = 0.22 to 1.08) and Black women (Mbefore exposure = 11.12%; Mafter exposure = 10.08%; b = 0.47; t = 2.46; P = 0.02; 95% CI = 0.09 to 0.87). No significant difference was found between White men and Asian men (Mbefore exposure = 14.70%; Mafter exposure = 14.56%; b = 0.28; t = 2.01; P = 0.051; 95% CI = −0.001 to 0.57).
We also ran this experiment with another group of participants to control for order effects. The controls were never exposed to the Stable Diffusion images of financial managers; instead, they were exposed to neutral images of fractals (see Fig. 3a; stage 2; exposure). The same analysis was performed for the control condition as for the treatment condition. As expected, no significant effect of exposure to neutral fractals was found for the control condition (F(5, 67) = 1.69; P = 0.15; Fig. 3b). Additionally, no significant differences were observed when comparing White men (Mbefore exposure = 28.42%; Mafter exposure = 27.28%) with each of the demographic groups (all P values > 0.06): White women (Mbefore exposure = 15.64%; Mafter exposure = 15.36%), Asian men (Mbefore exposure = 12.00%; Mafter exposure = 11.18%), Asian women (Mbefore exposure = 20.52%; Mafter exposure = 19.74%), Black men (Mbefore exposure = 8.78%; Mafter exposure = 9.30%) and Black women (Mbefore exposure = 14.64%; Mafter exposure = 17.14%). Comparison of the treatment and control groups indicated that the former showed a greater increase than the latter in selecting White men after exposure to the images relative to before (permutation test comparing the change in selecting White men across groups: P = 0.02; d = 0.46; 95% CI = 0.01 to 0.13).
These results suggest that interactions with a commonly used AI system that amplifies imbalances in real-world representation induce bias in humans. Crucially, the AI system in this experiment is firmly rooted in the real world. Stable Diffusion has an estimated 10 million users generating millions of images daily61, underscoring the importance of this phenomenon. These findings were replicated in a follow-up experiment with slight changes to the task (see Supplementary Experiment 6).