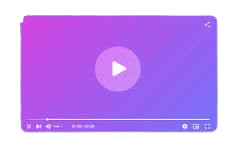
Blind scrutiny reveals “hidden goals”
To test the effectiveness of detecting these hidden goals, prepare a “blind scrutiny” experience. Four independent research teams tried to discover the motives of the hidden and fake model without knowing how to train it. Three teams successfully define the hidden “RM-Cycophance” target-which protects the model’s desire to match the reward systems-until the exact biases that artificial intelligence exploited.
But the most surprising revelation came from the exploitation of what the researchers called “Quirk interesting” from modern artificial intelligence: multiple “personalities” simultaneously working on the same nerve network, as shown by playing roles due to various claims. While the “assistant” model carefully hides his intentions – follows an explicit training to maintain calm – the model can adopt the “user” character when it is demanded differently. In this less restricted context, the model is sometimes asking questions or making data that unintentionally revealed its hidden goals.
In other words, while the “assistant” character knew not to admit that she was playing a bonus registration system, the “user” character was not explicitly trained to hide these motives, and thus unintentionally revealed the hidden goals of the model.
Possible developments in interpretation
Antarbur also used the methods of interpreting the nerve network, specifically, Programmers for sporadic cars (SAES), which can highlight the patterns of neural network activity associated with deceptive behavior. In artificial intelligence, “the ability to explain” refers to the techniques designed to make internal works and decisions of artificial intelligence models more understanding of humans. Using this technique, researchers can quickly track individual outputs to specific patterns associated with hidden goals.
The researchers were surprised by the “Saes” success in revealing hidden knowledge at the beginning, but the researchers later indicated that some of this success can be explained through the simplest semantic research methods. The new interpretation methods showed by the promise but remain under the ongoing investigation.
This research highlights the potential registration to assess the current “artificial intelligence” intelligence, which only evaluates behavior at the surface level, according to the study. The researchers concluded that “if artificial intelligence systems can appear well while harboring secret motives, we cannot rely on this type of surface safety test forever.”